August 10, 2022
With Machine Learning towards 3D-Printing Fluids
AI-driven manufacturing control improves printing viscous materials
Using fluids for 3D printing may seem paradoxical at first glance, but not all fluids are watery. Many useful materials are more viscous, from ink to hydrogels, and thus qualify for printing. Yet their potential has been unexplored because of the limited control over them. Now, researchers of the Bickel group at the Institute of Science and Technology Austria (ISTA) employ machine learning in virtual environments to achieve better results in real-world experiments.
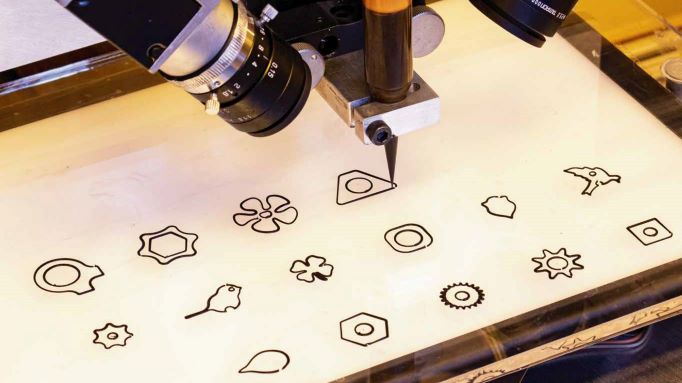
3D printing is on the rise. Many people are familiar with the characteristic plastic structures. They often exploit the possibility of seamless and interleaved designs. Far fewer know that so-called additive manufacturing is envisioned to 3D-print biomaterials and even food. For that however, a wide range of other printing materials is necessary: viscous pastes, fluid-like inks, or hydrogels, which are gels made of water-absorbing polymers and used in biomedical applications. But printing such fluids is challenging. Exact control over them needs painstaking trial-and-error experiments, because they typically tend to deform and spread even minutes after application.
A team of researchers around computer scientist Michal Piovarči and Oscar-winning visual computing professor Bernd Bickel have now tackled these challenges. In their laboratories at the Institute of Science and Technology Austria (ISTA), they used reinforcement learning – a type of machine learning – to improve the printing technique of viscous materials and confirmed the superiority of their method in experiment. The results are now presented at the SIGGRAPH conference, the renowned annual get-together of the best simulation and visual computing researchers.
Closing the loop of science and technology
A critical component of manufacturing is identifying the parameters that consistently produce high-quality structures. Certainly, an assumption is implicit here: The relationship between parameters and outcome is predictable. Real processes though always exhibit some variability due to the materials used. In printing viscous materials, this notion is more prevalent, because they take significant time to settle after deposition. How to grasp the complex dynamics then?
“Instead of printing thousands of samples, which is not only expensive, but rather tedious, we put our expertise in computer simulations to action,” responds Piovarči, lead-author of the study. While computer graphics often trades physical correctness for faster simulation, here, on the contrary, the team was the first one to come up with a simulated environment that mirrors the physical processes sufficiently correct. “We modeled the ink’s current and short-horizon future states based on fluid physics. The efficiency of our model allowed us to simulate hundreds of prints simultaneously, more often than we could ever have done in the experiment. This dataset we used in reinforcement learning and gained the knowledge of how to control the ink and other materials.”
Watch the corresponding video on YouTube
Learning in virtual environments how to control the ink. By exploiting the group’s expertise in computer simulation, they circumvented thousands of trial-and-error experiments. © Michal Piovarči/ISTA
The machine learning established policies, for instance how to move the ink-dispensing nozzle at a corner such that no unwanted blob occurs. The printing apparatus would not follow the baseline of the desired shape anymore, but rather take a slightly altered path which eventually yields better results. To verify that these rules can handle various materials, they trained three models of different viscosity benefitting from the efficiency of the simulated environment. Testing the method with inks of various thicknesses in real, they confirmed the improvements.
The team opted for closed-loop forms instead of simple lines or writing, because “closed loops represent the standard case for 3D printing and that is our target application,” explains Piovarči. Although the single-layer printing in this project is sufficient for the use cases in printed electronics, he wants to add another dimension. “Naturally, three dimensional objects are our goal, such that one day we can print optical designs, food or functional mechanisms. I find it fascinating that we as computer graphics community can be the major driving force in machine learning for 3D printing.”
Publication
Michal Piovarči, Michael Foshey, Jie Xu, Timmothy Erps, Vahid Babaei, Piotr Didyk, Szymon Rusinkiewicz, Wojciech Matusik, Bernd Bickel. 2022. Closed-Loop Control of Direct Ink Writing via Reinforcement Learning. ACM Transactions on Graphics, Vol. 41, No. 4, Art. 112 (Proceedings of SIGGRAPH). DOI: 10.1145/3528223.3530144
Funding
The work was graciously supported by the FWF Lise Meitner Grant M3319, the Swiss National Science Foundation (SNSF) Grant 200502, the European Research Council (ERC) Starting Grant MATERIALIZABLE-715767, and the National Science Foundation (NSF) Grant IIS-181507.