September 22, 2014
Tinkering evolution
IST Austria professor Krishnendu Chatterjee and collaborators propose concept of “regeneration process” in evolution • Evolution described as “tinkerer” modifying existing gene sequences rather than creating new ones • Study published in this month’s PLOS Computational Biology
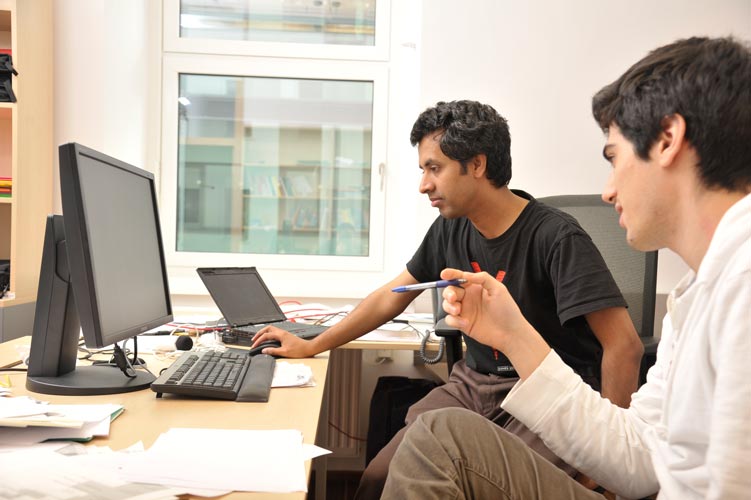
In a paper published this month in PLOS Computational Biology (DOI: 10.1371/journal.pcbi.1003818), Krishnendu Chatterjee, Professor at Institute of Science and Technology Austria (IST Austria), and his PhD student Andreas Pavlogiannis together with collaborators Ben Adlam and Martin A. Nowak from Harvard University investigate the timescale required for evolutionary innovation to arise.
A common question asked in evolutionary biology is how long it takes for an advantageous mutation to be established in a population. The authors approached evolutionary innovation from a different angle, asking how long it takes until a new function arises. For a sequence of amino acids with a certain length that undergoes adaptation, what is the expected time it takes until a new function is found? The authors here distinguish between two time requirements: polynomial time, where a new function is realistically achievable in the time course of evolution, and exponential time, where it is possible to get a new function in feasible time, but it is not likely and not expected in general.
During evolution, the starting amino acid sequence can change at each base. Some of these changes bring advantages, some disadvantages, and some have no effect at all. So for each sequence, there is a “landscape” of possible sequence variations, each with a different fitness level. With each mutation, the sequence hops from one point to another, exploring this fitness landscape. But how many jumps does it take on average to get to the target?
The authors used mathematical analysis of the underlying stochastic processes, as well as computer simulations to investigate three scenarios in a variety of fitness landscapes. When just one population evolves to find a new function in such landscapes, it requires exponential time to find it, making it unrealistically long until the innovation arises. When several populations evolve to find a new function, even the many searches going on at the same time don’t make it likely that the function arises at a realistic timescale. And even when one population evolves to find a new function and there are several ways in which this function can be achieved, it can still take exponential time to find it.
The results show that, mathematically, it takes unrealistically long to discover any meaningful innovation if a sequence explores the fitness landscape. But we do see new functions arising during evolution. How are these new functions then achieved? The authors propose a mechanism called “regeneration process”. It is based on the idea that evolution solves a problem more easily if it has already solved a similar one. Gene duplication and genome rearrangements provide a constant stream of sequences that are a certain number of mutations away from the target function. These sequences can be regenerated again and again. The researchers show that even without selection, a new function modified from these starting sequences can be reached within realistic, polynomial time with high probability, although not in all cases.
The proposed regeneration process formalizes several existing ideas about evolution. It ties in with the idea that duplication and genome rearrangement are major events that lead to the emergence of new genes. It also suggests that evolution can be seen as a “tinkerer” that plays around and modifies existing sequences rather than creating entirely new ones. The regeneration process is also related to a previous idea, suggesting that the starting sequence for exploring a fitness landscape must already have high fitness, or in this case, be close to the target function. The process also explains the emergence of orphan genes, new genes that arise through mutations in a previously non-functional sequence. The combination of these ideas breaks the barrier on time requirements for evolutionary innovation, making them possible on a realistic timescale.